Adverse media refers to negative or damaging information about individuals, organizations, or entities that can pose significant risks to businesses and other institutions. Such information can include news articles, social media posts, legal filings, and other public records that indicate potential involvement in financial crimes, corruption, or other illicit activities. The ability to effectively identify, collect, and analyze adverse media data is crucial for managing reputational, legal, and regulatory risks.
What is Adverse Media, and Why is It Important?
Adverse media refers to negative or damaging information about individuals, organizations, or entities that is publicly available through various sources such as news articles, social media, regulatory filings, court records, and other open sources. Adverse media can include information related to criminal activity, financial crimes, corruption, fraud, money laundering, terrorism financing, human trafficking, and other illegal activities.
Adverse media checks are important because adverse media monitoring can help identify potential risks and threats associated with individuals or entities. Financial institutions, for instance, use adverse media to screen clients and partners for potential money laundering or terrorist financing risks. Adverse media can also help companies to assess the reputation of their business partners or vendors and to ensure that they are not associated with any unethical or illegal activities.
Moreover, regulatory bodies such as the Financial Action Task Force (FATF) and the Office of Foreign Assets Control (OFAC) require financial institutions to conduct adverse media searches as part of their due diligence obligations. Failure to conduct proper adverse media screening can lead to severe penalties, reputational damage, and legal consequences.
What is Adverse Media in AML?
In the context of Anti-Money Laundering (AML), Adverse media refers to negative or unfavorable information about individuals, entities, or organizations that could indicate potential involvement in financial crimes, corruption, or other illicit activities.
Adverse media can include reports, articles, publications, or any publicly available information from reputable news sources, regulatory bodies, government agencies, or other reliable media outlets.
Financial institutions and businesses subject to AML regulations are required to conduct customer due diligence (CDD) and ongoing monitoring of their clients. Part of this process involves screening customers against various watchlists and databases, including adverse media sources. The purpose is to identify and assess potential risks associated with their customers or business partners.
By adverse media monitoring, financial institutions can mitigate the risk of being involved in illicit activities, avoid reputational damage, and comply with AML and KYC regulations.
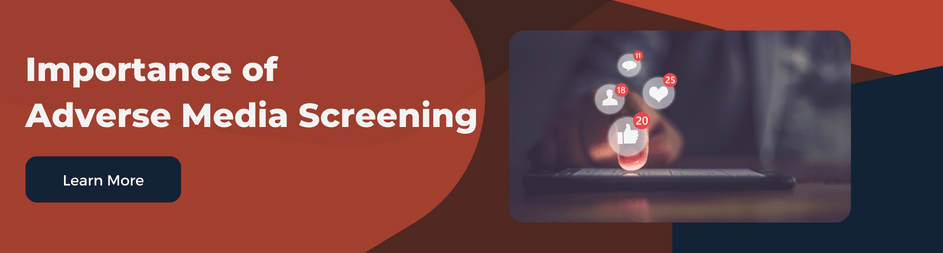
Adverse Media Risks for Financial Institutions
Financial institutions are particularly exposed to adverse media risks due to the nature of their business and the significant impact that negative information can have on their reputation, customer trust, and financial stability. Here are some of the adverse media risks that financial institutions should be aware of:
- Money Laundering and Terrorist Financing Risks: Financial institutions face significant regulatory and reputational risks if they become involved in money laundering or terrorist financing activities. Adverse media screening can help identify potential risks associated with customers, vendors, and business partners and allow institutions to take necessary steps to mitigate those risks.
- Compliance Risks: Financial institutions must comply with various regulations and laws related to anti-money laundering (AML), counter-terrorist financing (CTF), and other financial crimes. Adverse media search can help identify compliance risks related to customer due diligence (CDD), sanctions screening, and transaction monitoring.
- Reputation Risks: Negative media coverage related to financial fraud, corruption, or other illegal activities can severely damage a financial institution's reputation, customer trust, and market value. Adverse media screening and adverse media screening tools can help institutions identify potential reputational risks associated with customers, business partners, or vendors and take appropriate measures to manage those risks.
- Legal Risks: Financial institutions may face legal consequences if they fail to detect or report suspicious activities related to money laundering or terrorist financing. Adverse media screening can help institutions identify potential legal risks associated with customers, business partners, or vendors and take necessary steps to comply with regulatory requirements.
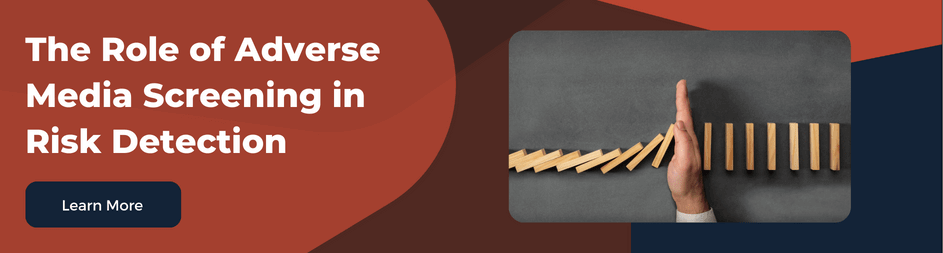
Methods and Techniques for Adverse Media Screening
Adverse media screening is the process of identifying and analyzing negative or damaging information about individuals, organizations, or entities that is publicly available through various sources. Here are some of the methods and techniques to do an adverse adverse media screening:
- Keyword Search: One of the most common techniques for adverse media screening is to use a keyword-based search to identify negative news articles, social media posts, regulatory filings, and other open sources. This involves identifying specific keywords or phrases related to financial crimes, corruption, fraud, money laundering, terrorism financing, and other illegal activities.
- Natural Language Processing (NLP): NLP is a technique used to analyze and understand human language. It involves using algorithms and machine learning to identify patterns and relationships in text data. NLP can analyze large volumes of adverse media data and identify potential risks and threats associated with individuals or entities.
- Data Aggregation: Adverse media data can be obtained from various sources, including news articles, social media, regulatory filings, and court records. Data aggregation involves collecting and consolidating this data from multiple sources to identify potential risks and threats associated with individuals or entities.
- Entity Resolution: Entity resolution is the process of identifying and linking information related to the same individual or entity across different data sources. This involves using algorithms and machine learning to match and merge data from multiple sources and to identify potential risks and threats associated with specific individuals or entities.
- Artificial Intelligence (AI): AI can be used to automate and streamline the adverse media screening process. This involves using machine learning algorithms to analyze large volumes of adverse media data and to identify potential risks and threats associated with individuals or entities. AI can also be used to improve the accuracy and efficiency of adverse media screening.
Overall, adverse media screening requires a combination of human expertise and technological tools to identify and analyze negative or damaging information about individuals, organizations, or entities. It is essential for financial institutions and other organizations to have effective adverse media screening methods and techniques in place to manage various risks associated with financial crimes, compliance, reputation, and legal consequences.
Collecting and Analyzing Adverse Media Data
Collecting and analyzing adverse media data is a complex and time-consuming process that requires a combination of human expertise and technological tools. Here are the steps involved in collecting and analyzing adverse media data:
- Data Collection: The first step in collecting and analyzing adverse media data is to identify relevant data sources and collect data from those sources. This involves using tools such as web crawlers, news aggregators, negative news screening tools, and social media monitoring tools to collect data from various sources.
- Data Cleansing: Once the data is collected, it needs to be cleansed and standardized to ensure accuracy and consistency. This involves removing duplicates, correcting errors, and standardizing formats.
- Data Analysis: Once the data is cleansed and standardized, it can be analyzed to identify potential risks and threats associated with individuals or entities. This involves using techniques such as keyword searches, NLP, data aggregation, entity resolution, and AI to identify patterns and relationships in the data.
- Risk Assessment: Once potential risks and threats are identified, they must be assessed to determine the level of risk and the appropriate response. This involves evaluating the severity of the risk, the likelihood of the risk occurring, and the potential impact of the risk.
- Reporting: Once the risks and threats are assessed, they must be reported to the relevant stakeholders. This involves creating reports and dashboards that clearly and concisely summarize the adverse media data and the associated risks and threats.
- Anti-Money Laundering Regulations: AML regulations require financial institutions to implement risk-based programs to detect and prevent money laundering, terrorist financing, and other financial crimes. Adverse media screening is an important part of these programs, as it helps to identify individuals or entities that may pose a risk.
- Office of Foreign Assets Control Sanctions: OFAC enforces economic and trade sanctions against targeted countries, individuals, and entities. Financial institutions must screen their customers and transactions against OFAC's sanctions lists, which include individuals and entities subject to adverse media.
- Know Your Customer (KYC) Requirements: KYC requirements require financial institutions to verify their customers' identity and understand their business activities and sources of funds. Adverse media screening is an important part of KYC, as it helps to identify any negative information or reputational risks associated with the customer.
- General Data Protection Regulation (GDPR): GDPR is a European Union regulation that governs personal data collection, use, and storage. Financial institutions and other organizations need to ensure that their adverse media screening programs comply with GDPR requirements, including obtaining consent from individuals for the use of their personal data.
- Fair Credit Reporting Act (FCRA): FCRA is a US federal law that regulates the collection, use, and disclosure of consumer credit information. Adverse media screening programs that use consumer credit information need to comply with FCRA requirements, including providing consumers with notice and obtaining their consent before conducting adverse media screenings.
- Danske Bank Money Laundering Scandal: Danske Bank, one of the largest banks in Denmark, was involved in a money laundering scandal that resulted in billions of dollars being laundered through its Estonian branch. The scandal was uncovered through adverse media screening, which revealed suspicious transactions and connections to high-risk individuals and entities.
- FIFA Corruption Scandal: FIFA, the governing body of international soccer, was involved in a corruption scandal that resulted in multiple arrests and indictments. Adverse media screening played a key role in uncovering corruption, as it revealed connections between FIFA officials and individuals and entities involved in bribery and money laundering.
- Wirecard Accounting Scandal: Wirecard, a German payments company, was involved in an accounting scandal that resulted in its collapse and bankruptcy. Adverse media screening played a key role in uncovering the fraud, as it revealed connections between Wirecard executives and individuals and entities involved in fraudulent activities.
- Huawei Sanctions Violations: Huawei, a Chinese technology company, was involved in sanctions violations that resulted in multiple indictments and fines. Adverse media screening played a key role in uncovering the violations, as it revealed connections between Huawei executives and individuals and entities involved in sanctions evasion.
- Harvey Weinstein Sexual Assault Scandal: Harvey Weinstein, a Hollywood producer, was involved in a sexual assault scandal that resulted in multiple criminal charges and lawsuits. Adverse media screening played a key role in uncovering the allegations, as it revealed a pattern of behavior and multiple victims over a period of years.
Regulations and Laws Related to Adverse Media
There are several regulations and laws related to adverse media that financial institutions and other organizations need to be aware of.
Adverse Media Examples and Case Studies
Here are some examples and case studies of adverse media that demonstrate the importance of effective screening and management of negative or damaging information:
Overall, these examples and case studies demonstrate the importance of effective adverse media screening and management in managing various risks associated with financial crimes, compliance, reputation, and legal consequences.
Sanction Scanner's Adverse Media Screening Software
Sanction Scanner provides an advanced adverse media screening software solution that enables financial institutions and other organizations to effectively identify potential risks related to crimes such as money laundering, terrorist financing, bribery, and drug trafficking.
Our globally comprehensive adverse media data is designed to support AML compliance processes, enabling businesses to perform adverse media checks in addition to PEP and sanctions screenings during customer onboarding. By utilizing our solution, companies can identify risks associated with their customer account opening processes and ensure that they comply with global regulatory requirements.
Our solution makes it easy for companies to perform adverse media checks with the customer's credentials, automatically checking comprehensive global sanctions, PEP, and adverse media data according to their risk levels. With Sanction Scanner, businesses can also perform adverse media checks via the API, web, or batch files, providing flexibility and customization options.